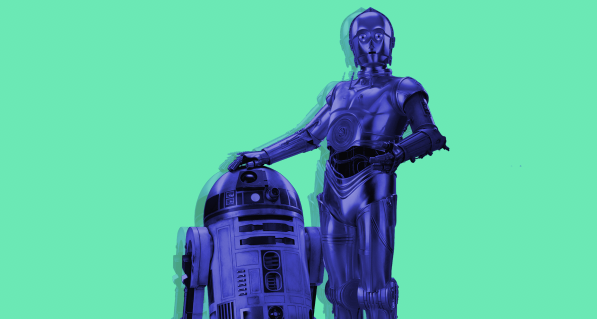
How many times in recent years have you heard the term artificial intelligence, or A.I for short, used in any of the following contexts?
AI is taking over our jobs! AI will strengthen the economy! AI is risky and unsafe! AI will drive huge efficiencies!
While there is considerable enthusiasm behind AI, we’ve only just scratched the surface of AI’s capabilities and benefits – and as we know, excitement can come with apprehension about whether we can avoid AI’s potential challenges and pitfalls.
We are in an era where business analytics and big data are driving more and more business decisions. Life sciences companies who can harness the power of AI and big data combined could gain a critical edge in the R&D race.
However, if you’re like me, you’ve been hearing AI pop up more and more at life science conferences and events, becoming the buzzword of choice from software vendors and industry experts at every opportunity. In the following article, we’ll examine what AI is at a high level for those that are less familiar with the subject and dive into what sort of real-world impacts it could have on clinical trials, more specifically trial master files.
What exactly is Artificial Intelligence (AI)?
AI is a branch of computer science capable of learning, finding patterns, and solving problems to provide insights from complex data - the underlying concept is that it can mimic human intelligence (I know, kind of creepy).
AI contains a variety of subdomains you may have heard of before, including; Machine Learning, Deep Learning, Neural Networks, Big Data, and Natural Language Processing.
What can be surprising to many is that Artificial Intelligence is already quite pervasive. Most of us are using it every single day, some of us without even realizing it! Did you know that Siri, Google Assistant, Cortana, and Alexa are all considered basic forms of AI?
According to biomedical scientist Perry Miller, we’ve been exploring artificial intelligence in medicine as early as the 70s. Since then, AI has been widely applied to the diagnosis, treatment, and measurement of predictive outcomes in clinical scenarios.
However, emerging technology could help us extend its use and provide mechanisms to recognize patterns that are vital to making decisions in life science research.
AI and its implications for the clinical industry
Let’s take a more in-depth look at how this came to be, and what it could mean for our future in the clinical space. CB Insights put it quite plainly; “the clinical trials market needs a makeover” based on the argument that trials are hugely inefficient, averaging nearly a decade, and costing companies billions of dollars.
From use in early disease diagnosis to matching patients with trials, monitoring patient progress, and data collection and management throughout the study - AI has numerous applications within the clinical space.
The main idea behind the research is that clinical problems yield to mathematical formulations. AI-driven diagnostics comb through a plethora of data and push this data through algorithms and machine-derived algorithms (Machine Learning) to suggest possible outcomes and identify any areas needing attention.
AI Adopters champion the technology for its potential to reduce resource expenditure and bring high-level optimization to administrative tasks.
We expect more AI projects to emerge and grow in the upcoming years as they capture the attention of large pharma companies. Part of the Johnson & Johnson family of companies, Jansenn Research & Development aims to speed up the drug delivery process using AI. In early 2018 Roche, the world’s largest biotech company acquired Flatiron Health to accelerate cancer research by using AI to capture structured and unstructured data.
On the other hand, skeptics who have not embraced AI argue that giving computer programs permission is dangerous for research (e.g. patient confidentiality). Many clinicians are thereby hesitant to allow AI become a large part of the decision-making process. In a 2018 study published by Emerj, nearly 50% of US companies responded that the healthcare industry “needs to be convinced further of ROI from Artificial/Machine Learning investments.”
AI has shown us promising results. Still, there is a need for further clinical trial case testing and development before emerging AI techniques find their ground in the everyday clinical setting.
AI and eTMF management
What does the fusion of AI and electronic Trial Master Files (eTMF) mean to the clinical industry?
Traditionally, AI-powered technologies have been used in healthcare to collect and structure data. The eTMF is no different, with AI providing deeper insight and coordination of clinical content held within the TMF, to help pave a broader understanding of the study storyline.
More specifically, AI can be used to extract meaningful information and meta-data from clinical study activity to structure and store this content within an eTMF in the most logical way. Unstructured data in the eTMF is a result of poor data exchange between sponsors and CROs. To alleviate this added source of stress, AI technology will be able to perform quality management of TMF content in alignment with the DIA’s TMF Reference Model and the sponsor’s designed TMF structure.
For instance, imagine that you have 2,000 unclassified, unstructured documents sitting in your eTMF. It would take a long time to go through and perform Quality Control (QC) manually. However, AI provides plenty of opportunities to improve efficiency and automate this process, saving you countless hours that you can put towards other research priorities. QC with AI would work in the following steps:
1. After sending a document to your CRO partner or sponsor, auto-classification would identify the document based on a pre-defined library selection
2. The system would then extract the data, and then extract further for each artifact at the Trial, Country, and Site level and then each sub-artifact field
3. It will then calculate and filter by confidence value to result in a final QC record, clearly showing researchers any sources of deviation
4. Any results showing low confidence scores can then be manually QC-ed by a researcher once the process is complete
When AI technologies integrate with the eTMF, trained algorithms can accurately process and extract data for faster and improved QC while reducing the room for human error. In the long run, AI integration will drive the eTMF’s evolution from a traditional repository of artifacts and documents to more of a data-driven source, improving our clinical decision-making.
En-route to more proactive clinical care
AI won’t replace our clinical researchers. We will always need people who have a holistic understanding of drug development and the complexities of human life. What AI can do is help as an intelligent assistance tool in making decisions, whether it be analyzing vast amounts of data or identifying patterns the human brain is incapable of recognizing.
Imagine you’re a clinician working with an AI-based Natural Language Processing technology during your patient meetings. With data on your study setting, the participants involved, and method of treatment – NLP, together with Machine Learning, can produce structured data and insights to further R&D. The system could interpret your conversations and interactions and provide you with deeper insights.
Final thoughts
AI can spark improvements in drug development by leveraging predictive analytics and bringing higher value resource contributions to the table.
Not only will AI improve our ability to innovate beyond our capacity to improve process flow, it will also help to make use of significant relationships between clinical datasets holding valuable information within the eTMF system. Optimizing this data and using it to make better clinical decisions would dramatically reduce the 10-15-year long process of drug development to deliver treatments to patients faster.
We certainly don’t have all the answers yet as to how AI will impact clinical research, but early indicators are promising. Further testing, system validation, and development of eTMF systems and AI will result in a better standard that our teams can get behind.
Share with us what you’ve learned about AI and clinical research!